Artificial Intelligence and Machine Learning: Lessons and Opportunities
Lessons learned from research and deployment of AI and ML solutions.
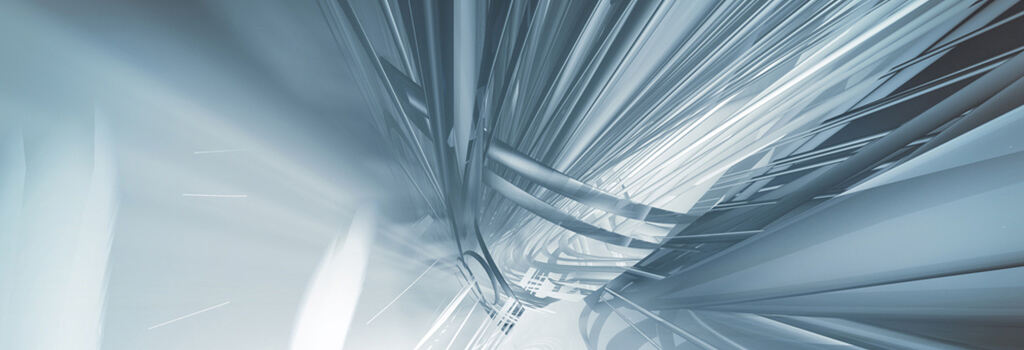
At this year’s Teradata Analytics Universe event, I shared some of the lessons I have learned over the years from my research and deployment of artificial intelligence (AI) and machine learning (ML) solutions, across multiple organisations, domains and industries.
During my talk, “Artificial Intelligence and Machine Learning - Lessons and Opportunities”, I explained that data science aims at resolving problems raised by customers, and in order to do that, data scientists use a number of technologies, such as ML, AI and deep learning. The focus is primarily centred on finding the right solutions to arising problems, spanning from the identity fraud to the prevention of equipment failure. To do that, data scientists must be capable of choosing the right tool amongst a myriad of them; because only the right tool will lead to the right solution.
Data science´s aim is to reach the right solution and not to just develop complex algorithms. For this reason, I pointed out some of the solutions that were outcome based:
During my talk, “Artificial Intelligence and Machine Learning - Lessons and Opportunities”, I explained that data science aims at resolving problems raised by customers, and in order to do that, data scientists use a number of technologies, such as ML, AI and deep learning. The focus is primarily centred on finding the right solutions to arising problems, spanning from the identity fraud to the prevention of equipment failure. To do that, data scientists must be capable of choosing the right tool amongst a myriad of them; because only the right tool will lead to the right solution.
Data science´s aim is to reach the right solution and not to just develop complex algorithms. For this reason, I pointed out some of the solutions that were outcome based:
- The first solution is represented by embedded sensors, which can predict disk failure one week before it happens, so that data loss can be prevented. This saves everybody´s work, it saves everybody´s time and also, it is another level of experience for the user.
- The second outcome-based solution comes from the customers´ complaints. Data scientists at Teradata worked with a large mobile manufacturer and, using NLP techniques, were able to identify merging complaints about products, by looking at the notes written by the customer service operators. The emerging problems were then confronted with the bill of materials of the supply chain to identify defects before these could become a media event and start to impact the brand.
- Another solution is to be found in the context of transportation networks. A problem tackled by data scientists at Teradata was the optimisation of the transportation networks. Here they looked at the pre-defined route to deliver the material from the manufacturer to the client. They did a “what-if analysis” on different transportation modalities (rail, trucks…) to come up with recommendations that helped the firm by avoiding some costs and providing the cheapest option.
Focused on the outcome
What does it mean for the business to solve a particular problem? Even by decreasing costs by just 1 or 2%, this can be translated into a massive impact on the business at bottom line.Data scientists at Teradata Consulting are helping shape the direction of AI and ML adoption. Teradata approaches these solutions as a team: architecture, foundation, engineering, cognitive design, they all rally around to solve this kind of problem, and this makes them much more efficient and successful – drawing upon the skillset of each other. It is a cross-functional agile team, where people blend in and blend out during the course of delivering the project.
When working at Amazon, one of the projects I worked on was AmazonGo, a new concept for grocery shopping. The concept of AmazonGo is a simple one: you walk in, you pick up one or more items and you walk out. There is no traditional check-out or self-service check-out, instead you are automatically billed with the products you have on you, as you leave the store. The concept of the AmazonGo store entails some ideas that go beyond just the technology side of it.
From a consumer side, it saves a lot of time, not having to wait in a queue to pay for goods. Plus, if you forgot something at the last minute, you will not stop the check-out process to go back and pick what you forgot. Therefore, AmazonGo offers a better customer experience.
Another aspect is the “no-empty shelves” event, which would be another missed opportunity for sales. In a normal store, it is not easy for store employees to realise a shelf if empty, unless they pass by it every few minutes. With AmazonGo, this doesn´t happen anymore, because the person in charge of refilling the shelves receives an alert telling them when shelf stock is low.
Possibly my favourite concept from working on AmazonGo was “Coupons 2.0”. In a normal store when you are given a coupon you either lose it within two minutes, or the product doesn´t appeal to you. With AmazonGo you could find yourself in this kind of scenario: you pick up a bottle of red wine and immediately receive a notification on your phone, advertising a package of pasta, which is on sale in another isle of the store. The idea is that consumers are advised on complementary products, ones that could help with meal options, while they are in store – meaning that the “coupons” are more valuable to the customer, right there and then – not after they have made their purchases for the day.
During my talk, I highlighted to the audience three personal lessons, which I had learned from the above insights I shared:
- When you are coming up with a proposal on what you want to do for AI and machine learning, there are so many benefits that you should look at, not just one. When Amazon announced the birth of AmazonGo, everyone thought they just wanted to reduce costs without thinking about how much better the customer experience would have become due to the more precise personalisation.
- Focus on the problem, not on tradition nor fashion. In movie recommendation for example, the tradition was collaborative filtering, the fashion is deep-learning.
- Get an environment set-up where you can build and deploy to production with AnalyticOps. It will not work the first time. You can´t avoid failure but what you can do is to minimize its impact. On average, once the model´s been trained, it takes about 5 moths before that goes into production. How do we reduce that to two weeks? With analytics (automatic deployment, alarm and monitoring) in operations – they have to have an impact on the bottom line of the business
To find out more information about my talk, you can watch the full presentation from Teradata Analytics Universe 2018 Virtual Experience. Register for free to watch, here.
Bleiben Sie auf dem Laufenden
Abonnieren Sie den Blog von Teradata, um wöchentliche Einblicke zu erhalten